Building Up Stable Data Science Teams
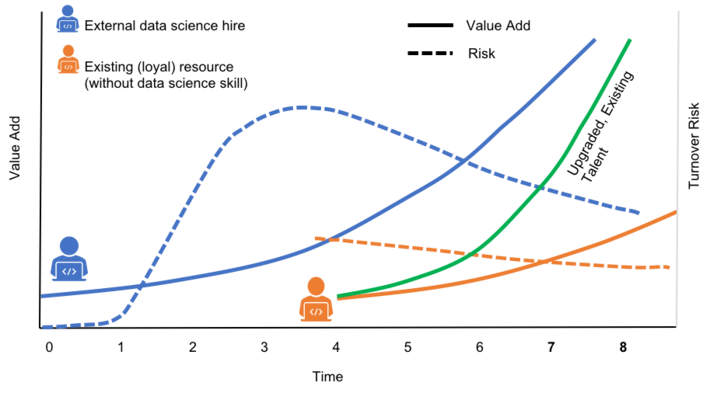
Navigating AI Transformation Challenges: Building a Lasting Data Science Team
Many large organizations face challenges in their AI transformation journey. Data science projects often take too long to complete or never make it to production. A major reason for this is the lack of a stable data science team, as high turnover rates plague the field due to high demand. To address this issue, data science managers and leaders typically focus on acquiring, retaining, and motivating top talent. However, this approach alone may not yield the desired results for many businesses. In this article, we discuss the various types of data science talent and how companies can minimize high turnover by training existing internal resources.
Three Types of Data Scientists
Data science talent in the market falls into three categories:
- Fresh graduates who have studied data science and machine learning but have not yet secured their first job,
- Experienced data scientists who have worked in the relevant industry for a few years, and
- Transitional talent with experience in other fields who have recently moved into data science.
Each category has its advantages and disadvantages. Hiring managers must decide based on their organization's immediate needs. If a project is already underway and requires a replacement, a data scientist with relevant industry experience may be the preferred choice. However, if an organization is just beginning its data science journey, a mix of all three types of data scientists might be more productive and cost-effective.
Uncovering Hidden Data Science Talent
Every organization has a hidden potential source of data science talent among its existing employees, such as business analysts, project managers, software developers, and data analysts. Many organizations have not focused on leveraging this talent by upgrading their skillsets through basic data science training.
While established data scientists with degrees in machine learning or AI might disagree, domain knowledge is just as crucial as technical data science expertise. A deep understanding of business processes and rules is essential to add value to the business, even if one has excellent data science and machine learning skills. Data science roles are highly cross-functional, and familiarity with an organization plays a critical role in success. Therefore, upgrading the skills of existing talent can be mutually beneficial for both the business and the individual.
Benefits for the Organization
Training existing resources can provide short-term benefits, such as:
- Improved communication among team members,
- Reduced need to hire new data scientists, and
- A sense of empowerment among existing talent.
In the long term, organizations can benefit from:
- Lower turnover rates, as existing talent is typically more loyal and has been with the company longer.
- Lower talent acquisition costs.
- Increased quality of data science innovation, as upgraded existing talent with deep business knowledge can more effectively produce quality innovation alongside their data science skills.
- Some of the upgraded talent may become passionate about data science, continue learning independently, and bring additional value to the organization.
This article was originally published on Data Science Central.