NextAI - Canada's Innovation Hub and Possible Machine Learning Application for Environment Monitoring and Protection
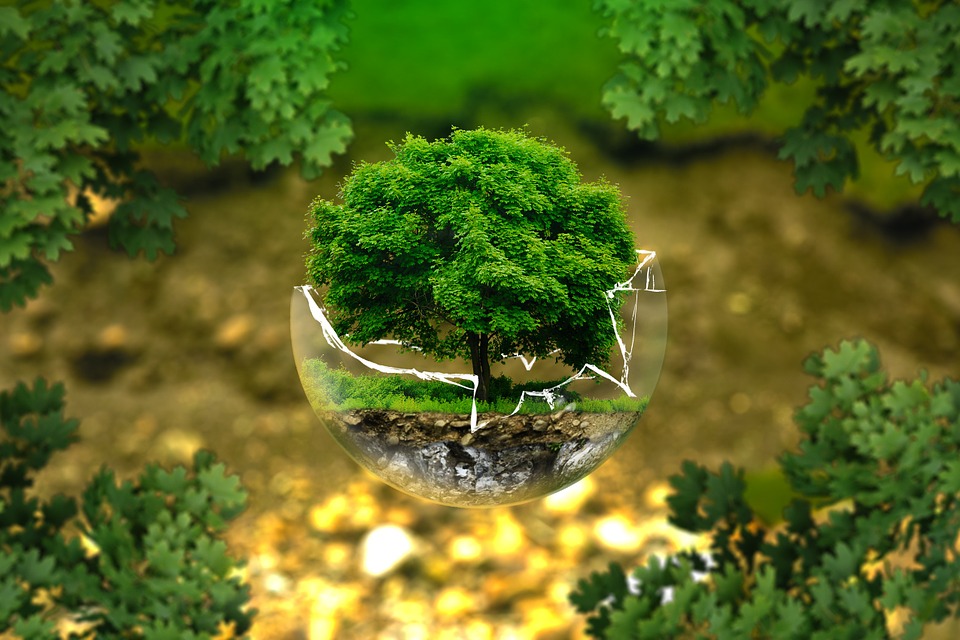
I, along with one of my former colleague at American Express Canada, have submitted a machine learning project proposal with NextAI. NextAI is a Toronto based idea accelerator with focus on articial intelligence and machine learning. It is backed by government (I guess, from both Ontario and federal goverment) and some of leading institutions in industry and academia.
In last quarter of each year, NextAI invites machine learning and artificial intelligence experts and people with background in business to apply for under this program. In first phase, applicants fulfilling the criteria are selected as finalists. In second stage, the finalists are encouraged to discuss their ideas and experience with one another and make a team by picking up one or more (finalist) partners. The teams have to submit their project applications to NextAI by briefly explaining their idea, its market value and how does it involve elements of article intelligence and machine learning. In third phase, a team may be called for a face-face interview in early January. The selected teams start working on their projects in early February, and obviously the team will get initial funding of $50,000.
After being selected as NextAI finalists, I and my friend discussed few potential machine learning project ideas with application in areas like human resource, fintech, telecommunication etc. Finally, we decided to go for a project with application to environment protection. Government of Canada has recently made strong commitment towards protecting environment by reducing greenhouse gases over coming years. We believe if we build a useful machine learning model, which could be used by governments and industry for greenhouse environmental control it would be a huge scientific contribution.
Modern, particularly big cities are facing huge population, business and traffic influx - building of new residential or business buildings, more production and supply of energy, increased number of cars, traffic and burning of fuel gas. These all factors contribute to outcome of increased greenhouse gases, heat and deterioration of air quality. Impact of contributing factors on outcome is not consistent over time and area of a city. It rather varies - high at office hours in key business and traffic zones, low on off days, high in residential areas on vacation days etc.
The problem is we know all these factors negatively impact our environment, but we can not measure this impact exactly. Furthermore, we do not exactly know how it fluctuates over time on different days of a week (and even in winter and summer).
We believe machine learning can help here through a model built by taking contributing factors into account. We hope to get training data from 1) city of Toronto (where we want to launch our pilot project and then replicate it elsewhere on provincial or federal level), 2) Torono's energy production and supplying companies, and real-time traffic data from Google maps. The main challenge would be to get training data labelled i.e. given input values at given time how do we know exactly the output metric, greenhouse gas or air quality? To sort this out we might need to collaborate with some established research group or come up with our own solution. The other option could be to treat it as an unsupervised machine learning problem.
The benefits of such a model are enormous; it could be used by city, provicial and federal authorities for urban planning and simulate how environment might be impact in future given new construction and expansion of cities. Energy production and supplying companies could use it to forecast demand against changes in weather, business and residential trends.